Ideas from a roundtable discussion
By Natalie Clark
Over the last five years, biologists have gained the ability to produce ever larger datasets at low costs, and have consequently started to more frequently collaborate with mathematicians, statisticians and bioinformaticians to determine the best ways to store, analyze, and interpret these data. As a trained biomathematician, I have learned how to integrate both computational and experimental techniques in my own research and so collaborating with experimentalists and computational scientists comes naturally to me, and I often find myself lending my statistical expertise to my colleagues. However, I often wonder how easy it is for pure experimentalists to reach out and collaborate with computational scientists and vice versa. Often experimentalists approach bioinformaticians with relatively simple tasks; how can we, as computational scientists, improve access to our own learning resources and make them more approachable for wet-lab scientists?
sometimes interdisciplinary collaborations never get off the ground due to a “language barrier”
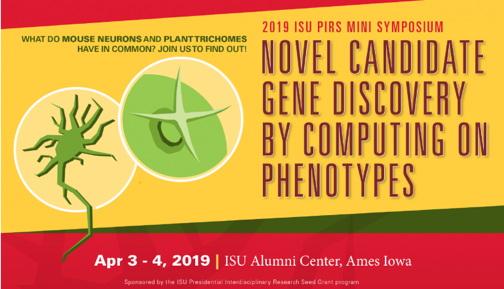
A first step might be to try and put experimental and computational scientists in the same space where they can converse, learn, and form collaborations. A recent example took place at Iowa State University, where I participated in a mini-symposium titled “Novel Candidate Gene Discovery by Computing on Phenotypes.”
This mini-symposium was made possible by the ISU Presidential Interdisciplinary Research Seed (PIRS) Grant awarded to Dr. Diane Bassham, Dr. Carolyn Lawrence-Dill, and Dr. Drena Dobbs from the Department of Genetics, Development and Cell Biology, and Dr. Matthew Ellinwood from the Department of Animal Science. The symposium was advertised as an interdisciplinary event where both experimental and computational scientists were encouraged to attend, with the idea of starting new conversations between them and creating new collaborations. Even the symposium’s image encouraged interdisciplinarity, comparing similarities in structures between mouse neurons and plant trichomes.
Concepts we think of as “simple” or “easy,” such as when to use a nonparametric test or the meaning of a p-value, may not be as readily comprehended by experimentalists
I found the two-hour long affinity group discussions at the symposium particularly rewarding. For these discussions, we were encouraged to suggest topics during the conference itself, following which the organizers picked four topics for roundtable discussions. Two of these topics focused on improving bioinformatics education and increasing interdisciplinary communication.
The attendees discussing interdisciplinary collaborations raised challenges with both starting such collaborations and figuring out how to facilitate them better. For instance, sometimes interdisciplinary collaborations never get off the ground due to a “language barrier”. People on either side speak in overly technical terms that the other side cannot understand. It is important that we, as computational biologists, understand that concepts we think of as “simple” or “easy,” such as when to use a nonparametric test or the meaning of a p-value, may not be as readily comprehended by experimentalists. On the other side, experimentalists should remember that many mathematicians and computer scientists might have not taken even a general undergraduate biology class.
As a group, we also agreed that, for a collaboration to be successful, it is important to take the extra time and effort to make sure that everyone understands the basic concepts before moving on to solving the complex problem. In addition to this, people on both sides need to be secure with their lack of knowledge and be unafraid to ask the “stupid questions” that might seem obvious to others.
Finally, we agreed that collaborations should feel natural and intuitive, and never forced. The suggestions raised by this affinity group are supported by numerous works done on the science of successful collaborations such as entries in the “Ten Simple Rules” series from PLOS Computational Biology (published in 2007, 2015, and 2017), and a 2017 study from the Institute for Collaborative Working on “Understanding the Psychology of Collaboration” which both summarize trends across effective collaborations.
I personally agree with these suggestions about facilitating interdisciplinary collaborations, but I also feel that difficulties with collaborations could be addressed much earlier by educating younger students about how to be interdisciplinary scientists.
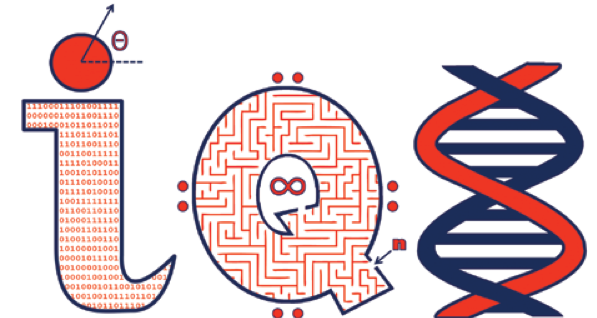
I was fortunate to have the opportunity to learn about interdisciplinary science at my undergraduate institution, the University of Richmond, as a member of the first cohort of its Integrative Inclusive Science program. The instructors of this program later published their findings that students in this interdisciplinary program were more likely to take additional math, physics, and computer science courses than students who did not participate in the class. Other educational institutions, such as North Carolina State University, Stanford University, Trinity College, Carnegie Melon, and others are also following this trend and offering their own integrative science programs at both the undergraduate and graduate level.
During the affinity group discussion at Iowa State, we also concluded that rather than trying to design a completely new major or curriculum, offering small, modular courses in interdisciplinary science would work better for undergraduate and graduate students that are already inundated with a large course-load. In my own experience the Integrative Inclusive Science minor I undertook as an undergrad took too much time away from other major courses, and students felt they had to choose between focusing on their major and participating in the program.
At the symposium, we participants also envisioned that these modular classes should be co-taught by a biologist and a statistician and cover topics such as biostatistics, non-parametric and non-linear statistics, information theory, machine learning, basic mathematical modeling, and basic programming. Such modules should be also be geared towards first- and second-year students and not just students that have already declared a major as we believe education in these cross-disciplinary topics should start as early in the career as possible. Finally, we felt that while resources are available that offer lessons on these topics, we found them inaccessible for students (i.e. are poorly advertised or behind a pay wall) or lacking in the lessons themselves (i.e. courses assume a certain degree of background knowledge that not all students have).
In conclusion, I think that more workshops and conferences should incorporate discussion groups focused on interdisciplinary education and collaborations so that we can continue to obtain feedback, improve pre-existing programs, and develop new solutions.
From my personal experience, and from what I heard from others at the 2019 PIRS Mini-Symposium, I strongly encourage young scientists interested in interdisciplinary topics to start learning now. Take a summer course in programming or sit in on a course outside your major just because it sounds interesting, not because it is a requirement. I also encourage those scientists in interdisciplinary collaborations to start more conversations. We should introduce our broader professional networks to our collaborators and help our fellow scientists find new collaborations.
Through better education and networking, we can improve resources for all scientists so that as technology continues to evolve, datasets keep getting larger, and biological problems become ever more complex, we can provide the interdisciplinary solutions that are so sorely needed.
Dr. Clark is a USDA-NIFA Postdoctoral Fellow at Iowa State University. She thanks Dr. Diane Bassham, Dr. Carolyn Lawrence-Dill, Dr. Drena Dobbs, and Dr. Matthew Ellinwood for their thoughts and comments on this article Dr. Clark is a USDA-NIFA Postdoctoral Fellow at Iowa State University.